In the world of artificial intelligence, data is king. However, not all data is created equal.
The quality of data used in AI systems can significantly impact their accuracy and effectiveness - a fact often overlooked in the rush to implement AI solutions.
In this article, we'll explore the importance of data quality in AI systems, how it affects AI outcomes, and provide insights on improving data quality for better results.

Understanding Data Quality in AI Systems
There's much more to data quality than just having accurate data.
It’s a critical factor in AI systems, encompassing several dimensions, each crucial to the overall effectiveness of AI systems.
But what exactly is it? Let's break it down further.
What is Data Quality?
Data quality refers to the condition of a set of values of qualitative or quantitative variables. In simpler terms, it's about how well your data represents the real-world scenarios you want your AI to understand and learn from.
High-quality data is clean, accurate, complete, and relevant to your specific needs.
The Five Dimensions of Data Quality
When discussing data quality, we often consider five main aspects:
- Accuracy: How accurately does the data mirror the real-world scenario it portrays?
- Completeness: Are there any crucial pieces of data missing?
- Consistency: Does the data maintain consistency across all sources and over time?
- Reliability: Can the data be relied upon as a trustworthy source?
- Timeliness: Is the data current and readily available when required?
Each of these dimensions plays a vital role in ensuring the quality of data used in AI systems; ensuring these criteria are met can significantly improve the accuracy and effectiveness of your AI system.
The Critical Role of Data Sources and Integration
Data sources are the lifeblood of AI systems. They provide the raw material that AI uses to learn, make decisions, and perform tasks. That said, their quality and reliability can vary significantly.
How Data Sources Affect Quality
Needless to say, where you get your data from can greatly influence data quality.
For instance, data from a reliable government database is likely to be more accurate and complete than data scraped from social media.
Therefore, choosing the right data sources is always a critical first step.
The Importance of Effective Data Integration
Once you have your data, it's not enough to just dump it into your AI system - you need to integrate it effectively.
This process is crucial for maintaining consistency and reliability, which, as you’ll remember, are two key dimensions of data quality.
Data integration involves combining data from different sources and providing users with a unified view of these data. Without one, your AI system may end up with conflicting or redundant data, leading to poor performance and inaccurate results.

Validating Data for AI Accuracy
Data validation is vital if you want to avoid inaccurate predictions or decisions from your AI system.
If the data isn’t checked for accuracy and consistency, your AI system might be learning from incorrect or inconsistent data.
Techniques for Data Cleaning and Preprocessing
Data cleaning and preprocessing are key to data validation.
Data cleaning involves identifying and correcting errors in the data, such as missing values or outliers, while preprocessing involves transforming the data into a format that the AI system can easily understand and learn from.
Both these steps help ensure that the AI system is learning from accurate, consistent, and relevant data.
The Role of Human Oversight in Data Validation
While automated tools can do much of the heavy lifting in data validation, human oversight remains indispensable. That’s because humans can spot patterns or issues that automated tools might miss. We can also make judgment calls about the relevance or appropriateness of certain data for the AI system.
In this way, human oversight helps ensure that the data used in AI systems is not just technically correct but also meaningful and useful.
How Poor Data Quality Affects AI Systems
Poor data quality can have a majorly detrimental impact on AI systems.
AI systems learn from the data they're given; therefore, if the data is inaccurate, incomplete, or inconsistent, the AI system's learning will be flawed. And this can have serious consequences.
Examples of How AI Could Fail Due to Data Issues
Inadequate data quality can cause AI system failures, resulting in severe real-world consequences.
For example, an AI system used for medical diagnosis might make incorrect diagnoses—and lead to fatalities—if it's trained on inaccurate or incomplete medical data. Similarly, an AI system used for financial forecasting might make poor investment decisions if it's trained on inconsistent or outdated financial data. In turn, this can result in major financial losses.
The Cost of Inaccurate Data in AI Projects
Indeed, the cost of inaccurate data in AI projects can be high. Not only can it lead to poor outcomes, but it can also waste valuable resources.
Correcting data errors after the fact can be time-consuming and expensive, too, highlighting the importance of getting data quality right from the start.
Business Intelligence and Data Accuracy
Business intelligence is all about making informed decisions based on data. Therefore, if the data is inaccurate, the decisions made could be flawed, leading to poor business outcomes, such as lost revenue or missed opportunities.
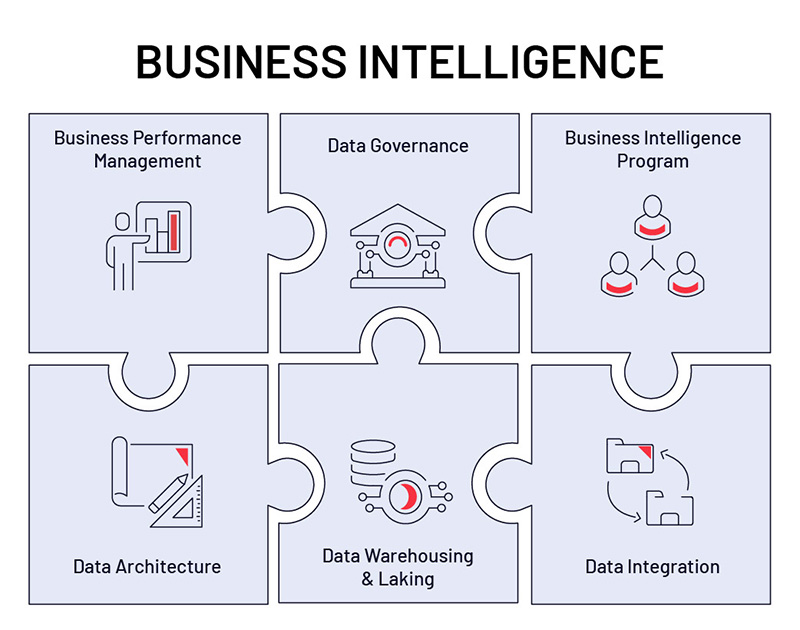
The Impact of Data Quality on Business Decisions
Nowadays, data is at the heart of all business decisions. When data is high-quality, it provides businesses with precise insights, enabling better decision-making and giving a competitive edge to innovate rapidly and outperform competitors.
Conversely, poor data quality can lead to misguided strategies being implemented.
It really can go either way.
Best Practices for Ensuring High-Quality Data in AI
Maintaining top-notch data standards for AI isn't a one-off job. It requires consistent attention and a strategic approach. Here are some key practices to keep in mind:
- Set up a robust data governance framework to ensure consistency and reliability of data across the organization
- Conduct regular data quality audits to identify and rectify data quality issues in a timely manner
- Implement continuous improvement processes over time
- Use automated tools for data quality assessment
- Involve domain experts in data validation
The Future of AI and the Increasing Importance of Data Quality
With every passing day, AI systems are becoming increasingly complex and sophisticated. What’s more, they’re being used for more critical applications where the cost of errors can be high.
Therefore, as AI continues to evolve, data quality will only become more important.
Ethical Considerations and the Role of Data Quality
Ethical implications are one facet that will take on increasing prominence in the context of AI Data quality. Poor data quality can lead to biased AI outcomes, which can be unfair and discriminatory. Ensuring high data quality is, therefore, not just a technical issue but also an ethical responsibility.
This is why, as we prepare for the next wave of AI innovations, we must prioritize data quality. This will help us build AI systems that are not only accurate and effective but also fair and trustworthy.
Conclusion
Amid the unstoppable rise of AI, it's time to put data quality at the forefront of your AI strategies. Failure to do so can impact systems’ accuracy and effectiveness, and can even have ethical implications.
By prioritizing data quality, we can harness AI's full potential and drive meaningful progress in our businesses and society.
At Further, we help businesses create strategic advantage with AI. Learn more about our AI solutions today.