Data driven experience optimization is not just a buzzword; it’s a strategic necessity for any business focused on growth. You’re about to discover how leveraging data can shape every facet of the user experience, from the first click to the final transaction. This article delivers no-fluff, actionable insights on optimizing engagements and outcomes, with practical examples of A/B testing, personalization, and predictive analytics that you can apply today.
Key Takeaways
- Data-driven mapping and personalization of user journeys utilizing historical and real-time data can significantly enhance customer engagement and satisfaction.
- A/B and multivariate testing, guided by statistically significant data and sound test hypotheses, are essential tools for optimizing user experiences and driving business objectives.
- Continuous experience optimization is an iterative process involving machine learning for predictive analysis, scaling personalization efforts, and creating feedback loops to refine user engagement strategies while navigating data protection standards.
Harnessing Data to Enhance User Journeys
In the business world, customer journey is at the heart of every decision, interaction, and touchpoint, each contributing to the user’s experience. But how can we truly understand this journey? The answer lies in harnessing and analyzing historical data. Examining past user interactions with your business can provide valuable insights into the different stages of the user journey, which can then be used to enhance engagement and customer satisfaction.
Beyond understanding, data also plays a crucial role in personalizing the user journey. Leveraging big data opens up the possibility of personalization on a scale previously unimaginable. By tailoring content, interactions, and recommendations to individual users, businesses can create unique experiences that not only engage customers but also set them apart from the competition.
Mapping the User Journey
Diving into the user journey begins with data-driven mapping. Businesses can track customer interactions from the initial website visit to post-purchase support using analytics tools. This tracking helps guide improvements in customer satisfaction and provides data-driven insights into the user journey.
Creating a customer journey map visualizes this process, helping pinpoint areas requiring enhancements for a better customer experience. Identifying these critical touchpoints within the user journey allows businesses to gain a deeper understanding of consumer interactions with their brand. This holistic view of the customer experience enables businesses to optimize the overall user journey and amplify user engagement.

Identifying Pain Points
Just as a journey has its highlights, it also has its hurdles. Identifying these pain points in the user journey is essential for businesses striving for seamless customer experiences. However, quantitative data alone might not capture the nuances of user experience. Therefore, qualitative insights become key in this process, delving into the motivations, thoughts, and attitudes of users.
A comprehensive view of user difficulties can be formed through the analysis of customer feedback and studying user interactions. By striving to understand user behavior, this understanding allows organizations to tailor solutions to specific issues, thereby enhancing the overall customer experience and boosting user engagement.
Personalization Tactics
Once the user journey is mapped and pain points identified, the next step is personalizing the user experience. Personalization enhances user engagement and can significantly increase retention rates. Businesses can create a unique and engaging user journey by tailoring content and offers to users based on their behavior and preferences.
Achieving effective personalization, however, requires systematic testing and refinement. Iterative testing cycles and A/B testing play a crucial role in determining the most successful personalization tactics. Businesses can identify successful personalization elements, leading to enhanced user engagement and conversion rates, by experimenting with different strategies and continuously refining them based on user responses.
The Role of A/B and Multivariate Testing in Optimization
As we delve further into the world of data-driven optimization, we encounter two powerful tools: A/B and multivariate testing. A/B testing, also known as split testing, involves comparing two versions of a webpage or app, while multivariate testing, also known as a multivariate test, compares different content combinations across pages to find the best conversion rates.
These testing methods are instrumental in determining the best user experiences for achieving specific business objectives. Businesses can make data-driven decisions to enhance overall performance by measuring the impact of different content variations on user behavior and performance metrics.
Crafting Test Hypotheses
The foundation of successful A/B testing lies in crafting sound test hypotheses. Formulating a hypothesis involves defining a problem based on observations and proposing a solution to test experimentally. A good hypothesis pinpoints how a change will affect customer behavior and potentially increase conversion rates, guiding the testing process and impacting conversion rate optimization.
However, it’s not enough to have just one hypothesis. Multiple ideas should be brainstormed and ranked by potential impact. The hypothesis with the highest likelihood of success should be chosen for testing. Proper sample size calculations are crucial to ensure the test is adequately powered to detect true differences in conversion rates.
Executing Tests and Analyzing Results
Once the test hypothesis is crafted, the next step is executing tests and analyzing results. A/B testing allows businesses to test changes to one element at a time, identifying the specific cause of any changes in user behavior. In contrast, multivariate testing can be used to test changes to several elements simultaneously, understanding their combined effect.
The power of these tests lies in the quantitative data they provide. Businesses can establish whether test outcomes are statistically significant by gathering data from A/B testing and user surveys. This analysis not only validates the tests but also measures the business impact of A/B testing by comparing the additional revenue generated by the test against the costs of running the test.
Learning from Test Outcomes
The learning journey doesn’t end with test execution. The outcomes of A/B testing provide valuable insights that can guide future testing strategies. Key performance indicators such as conversion rate, average order value, and revenue per visitor are essential for assessing the impact of A/B testing on revenue and optimizing future tests.
The choice between multivariate testing and iterative testing should be guided by learnings from past test outcomes. Considerations for resource availability, variable complexity, time sensitivity, and risk tolerance all factor in, ensuring businesses make the most informed decisions for their user experience optimization efforts.
Leveraging Machine Learning for Predictive Analysis
As we continue to explore data-driven optimization, we cannot overlook the transformative power of machine learning. Machine learning models can:
- Predict user behavior and intent by analyzing historical data
- Provide a dynamic tool for businesses to proactively address potential issues
- Enhance customer experiences
The power of machine learning extends beyond prediction. Businesses can understand customer behavior, preferences, and needs on a deeper level by integrating data analytics and artificial intelligence. This understanding allows businesses to deliver personalized experiences on a large scale across various channels, creating a system of continuous experience optimization.
Predictive Modeling Techniques
Predictive modeling techniques like linear regression, decision trees, and neural networks form the backbone of data-driven optimization. Linear regression estimates relationships between variables, facilitating marketing analysis such as the impact of advertising spend, sales forecasts based on pricing and promotions, and market segmentation.
Decision trees, on the other hand, excel at handling numerical and categorical data, managing nonlinear relationships, and segmenting audiences. Neural networks, especially deep learning models, are adept at processing large and complex datasets. These techniques play a crucial role in customer segmentation, recommendation systems, and sentiment analysis.
Real-Time Data Utilization
The power of data-driven optimization extends beyond historical data. Real-time analytics can inform immediate adjustments to improve user experiences, facilitating rapid changes based on current user interactions and providing valuable data insights.
Personalization of customer experiences is enhanced through the analysis of real-time data, enabling a more tailored interaction as user behavior is observed. Businesses can create real-time personalization feedback loops by integrating data from all customer interaction touchpoints into a centralized system and employing AI and automation.
Protecting Sensitive Information
As businesses delve deeper into data-driven optimization, safeguarding sensitive information becomes paramount. Major data protection laws like:
- GDPR
- HIPAA
- GLB Act
- FTC Act
- CCPA
mandate the protection of sensitive information, each with significant repercussions for noncompliance.
Organizations are required to implement comprehensive data privacy policies to ensure compliance. This includes:
- Obtaining user consent for data collection
- Adhering to privacy regulations
- Regular training for employees involved in data processing to ensure they are aware of data protection standards and the importance of compliance.
Key Metrics for Measuring Experience Optimization
As businesses embark on their journey of data-driven optimization, it becomes crucial to measure the effectiveness of their optimization strategies. Key metrics such as conversion rate, user engagement, and business impact metrics help assess the success of these efforts.
These metrics not only provide a quantitative measure of success but also serve as a guide for future optimization efforts. Businesses can ensure they are on the right track and make necessary adjustments to further enhance their user engagement strategies by consistently monitoring these metrics.
Conversion Rate Metrics
Conversion rate and click-through rate are two key metrics used to evaluate the success of optimization efforts. The conversion rate is calculated by dividing the number of visitors who completed a desired action by the total traffic during a specified period. This metric provides insight into the effectiveness of a business’s optimization strategies.
Click-through rate (CTR), on the other hand, is computed by dividing the number of clicks on a link by the total number of times the link was shown. This metric serves as an indicator of initial user engagement and interest. Together, conversion rate and CTR provide a comprehensive view of user engagement and the effectiveness of optimization efforts.
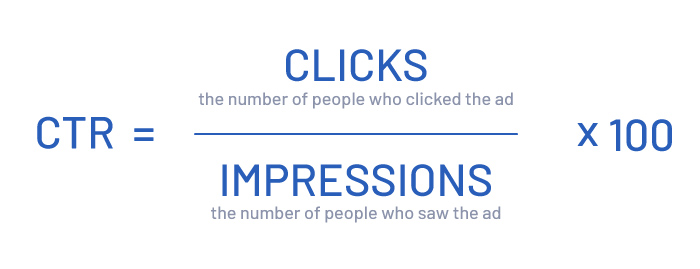
Engagement and Retention Metrics
Engagement and retention metrics provide additional insights into user interaction and engagement. Some examples of these metrics include:
- Unique visitors
- Return visitors
- Time spent on page
- Bounce rate
These metrics give businesses a holistic view of user engagement.
Average session duration and events per session are indicative of how engaging and interactive the product is for users. The retention rate reflects the percentage of users who return after their initial visit, measuring the stickiness of a website or product. By monitoring these metrics, businesses can continuously refine their strategies to enhance user engagement.
Business Impact Metrics
Beyond engagement and conversion, businesses must also look at the monetary benefits of their optimization efforts. One way to do this is by tracking revenue metrics like average revenue per user (ARPU). By dividing monthly recurring revenue by the number of paying users, businesses can understand the monetary benefit derived from their data-driven optimization efforts.
Monitoring these metrics allows businesses to assess the overall impact of their optimization efforts. Armed with this knowledge, they can make strategic decisions to further enhance their user engagement strategies and maximize revenue.
Implementing Continuous Experience Optimization
The journey towards data-driven optimization is not a one-time event; it’s a continuous process. This process involves iterative testing, scaling personalization efforts, and establishing feedback loops for ongoing improvement of the user experience.
Businesses can achieve ongoing improvements in user experience by persistently applying the principles of iterative testing and feedback loops. Continuous experience optimization allows businesses to routinely reassess and apply data-driven insights, ensuring their strategies remain effective and relevant in an ever-changing landscape.
Iterative Testing Cycles
A core component of continuous experience optimization is iterative testing. Continuous A/B testing provides a stream of recommendations that can be utilized for fine-tuning performance, especially with regards to evaluating digital marketing assets.
Iterative testing processes, such as A/B testing, analyze results and establish a cycle of testing that leads to solutions enhancing website conversion rates. Decentralized testing further aids in the scalability of personalization efforts, providing the ability to quickly adapt to user feedback and changing market trends.
Scaling Personalization Efforts
Scaling personalization efforts is a critical aspect of continuous experience optimization. This involves identifying various touchpoints, from website navigation to email marketing, where personalized experiences can be delivered. By integrating personalization across these touchpoints, businesses can create more engaging and tailored user experiences.
Data-driven insights form the backbone of these efforts, providing the necessary guidance to tailor experiences that resonate with diverse customer profiles. Proactive and ongoing refinement of personalization techniques is crucial as it allows businesses to stay aligned with evolving customer preferences and enhance user engagement continuously.
Feedback Loops and Adjustments
Creating feedback loops is essential for data-driven decision-making in continuous experience optimization. Businesses can create real-time personalization feedback loops by integrating data from all customer interaction touchpoints into a centralized system.
Iterative testing cycles in continuous optimization involve monitoring and analyzing outcomes, then making data-informed adjustments based on feedback before proceeding. This constant fine-tuning ensures businesses stay on top of their user engagement optimization efforts and continue to deliver enhanced customer experiences.
Navigating Challenges in Data Driven Optimization
While the benefits of data-driven optimization are plentiful, the journey is not without its challenges. From over-reliance on data stifling creativity to misinterpretation of data leading to incorrect decisions, businesses often encounter hurdles on their path to successful data-driven optimization.
However, these challenges can be navigated. Businesses can ensure data is accurately interpreted and implemented in design by fostering a synergistic approach between data scientists and UX designers. Additionally, collaboration with skilled partners allows organizations to allocate digital team resources towards enhancing business outcomes.
Balancing Quantitative and Qualitative Data
Balancing quantitative and qualitative data is a critical aspect of data-driven optimization, especially when aiming to collect data effectively.
Quantitative research focuses on collecting numerical data to:
- Identify patterns
- Make predictions
- Generalize findings
- Benchmark
- Calculate return on investment
However, quantitative data alone might not capture the nuances of user experience. Therefore, qualitative data becomes key in this process, delving into the motivations, thoughts, and attitudes of users. Businesses can gain a more comprehensive understanding of user behavior and create experiences tailored to user needs by combining both quantitative and qualitative data.
Sample Size and Statistical Significance
Another challenge in data-driven optimization is ensuring adequate sample sizes for accurate conclusions and validation of test results. Quantitative research with large sample sizes can result in statistically relevant data. A sufficient sample size provides a more impersonal and broader representation of audience behavior.

Statistical significance is better assured when conclusions are based on data derived from adequately sized samples. Careful determination of sample size is crucial for validating test results and achieving statistically significant results within user engagement optimization initiatives, and this can be achieved through proper statistical analysis.
Adapting to Evolving Data Protection Standards
In today’s digital age, data protection is paramount. Businesses must stay up-to-date with data protection regulations to ensure all user engagement optimization practices comply with current regulations.
Organizations are required to implement comprehensive data privacy policies to ensure compliance. This includes:
- Obtaining user consent for data collection
- Adhering to privacy regulations
- Regular training for employees involved in data processing to ensure they are aware of data protection standards and the importance of compliance.
Summary
In conclusion, data-driven optimization is a powerful tool that businesses can leverage to understand and enhance the user journey, personalize customer experiences, and drive user engagement. By harnessing historical data, conducting A/B and multivariate testing, leveraging machine learning, and implementing continuous experience optimization, businesses can create tailored experiences that not only engage customers but also set them apart from the competition. However, it’s crucial for businesses to balance quantitative and qualitative data, ensure adequate sample sizes, and stay updated with evolving data protection laws to navigate the challenges in data-driven optimization successfully.
Ready to improve your user experience with data-driven optimization? Contact Further to learn how.
Frequently Asked Questions
What is data-driven optimization?
Data-driven optimization involves using historical data, A/B testing, and machine learning to enhance the user journey, personalize customer experiences, and drive user engagement. It is a powerful process for improving business outcomes.
How can businesses personalize the user journey?
To personalize the user journey, businesses should analyze historical data, identify user pain points, and implement personalization tactics through A/B and iterative testing. This approach will help create a more tailored experience for users.
What is A/B testing, and how does it help in optimization?
A/B testing is a way to compare two versions of a webpage or app to see which one performs better, helping to optimize the user experience and achieve business objectives by measuring the impact of different content variations on user behavior and performance metrics.
How can machine learning aid in data-driven optimization?
Machine learning models can aid in data-driven optimization by predicting user behavior and intent and enabling real-time personalization, ultimately enhancing user experiences. By analyzing historical data, machine learning can help identify patterns and trends that lead to more effective decision making.
What challenges might businesses face in data-driven optimization, and how can they navigate these?
To navigate challenges in data-driven optimization, businesses should foster collaboration between data scientists and UX designers, balance quantitative and qualitative data, ensure accurate sample sizes, and stay updated with data protection laws. This can help address concerns related to over-reliance on data, misinterpretation of data, and compliance with evolving data protection laws.