According to Statica, companies spend $75 billion on marketing research to better understand their customer’s sentiments, needs, and experiences. But, most companies already have terabytes of open-ended customer feedback data collected through call center phone recordings, chat transcripts, emails, and social media. This post explains how call center data can be used to do the following:
- Generate insights to help brands more efficiently handle customer needs
- As a substitute for traditional market research to gauge customer concerns to improve their experience
- Improve brands’ Interactive Voice Response (IVR) to direct customer calls and provide needed information
- Leverage an understanding of customer needs as an ideation source for new product or service ideas
What are the benefits of leveraging call center data?
Harvesting customer feedback data can provide critical insights that lead to improved customer experiences, new products or services, and reduced interaction costs.
Instead of relying on anecdotal information or finding room in your budget for expensive primary research like surveys or focus groups about theoretical ‘target audiences’, companies can obtain the fact-based understanding needed to provide well-informed customer experiences that generate brand loyalty, higher revenue, and reduced customer service expenses.

What are the challenges involved with leveraging call center data?
Even though this data represents one of the richest sources of customer experience information, most companies don’t take full advantage of it. Why? Because it’s complicated. Businesses wanting to harness customer feedback data requires marketing expertise, technological sophistication, data science capabilities, and protection of Personally Identifiable Information (PII).
Finding the right team with experience and expertise to unlock call center data can feel overwhelming. As if that wasn’t hard enough, companies must also connect the dots with other data and company strategy. According to the Wall Street Journal, partnering with a strong and innovative IT partner can make all the difference. Consultancies like Further posses the technical skills along with deep domain expertise to help companies get the most out of their call center data.
Artificial Intelligence (AI) makes customer feedback data accessible
Advances in AI unlock previously inaccessible data sources, including call center data, to provide real-time answers to questions like these:
- Why do customers leave for the competition?
- How can companies address customer concerns before expensive customer service interactions?
- What other products and services do customers want?
However, as with any cutting edge technology, extracting insights from unstructured data like customer service phone call recordings presents many challenges.
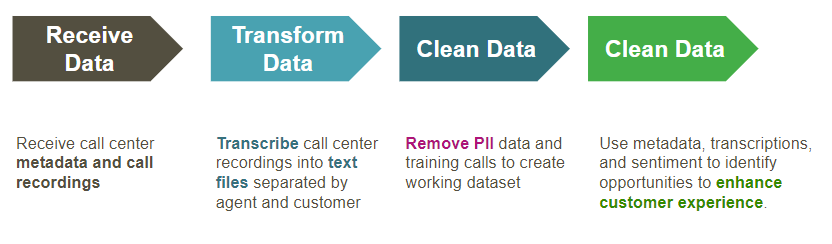
Data pipelines and Machine Learning (ML) models
Unlocking this treasure trove of data begins by building data pipelines and machine learning models to mine and understand customer interactions. A data pipeline transforms unstructured data like customer service phone call recordings and chat transcripts into a form easily consumable by data scientists and ML models.
As a Premier Google Partner, Further employs the power of Google Cloud Platform for our AI needs. Google Cloud allows us to harness the tremendous computing power of Google’s backbone, the same infrastructure that runs YouTube, Google Search, Gmail, etc. We can quickly deploy massive computing power in isolated, self-contained projects using the best available technology.
Companies often begin with a low risk proof-of-concept to better understand the process and their data, while more mature companies may put in place a complete end-to-end solution.
One critical aspect of the data pipeline is protecting customer privacy.
Privacy Protection
Privacy protection is key both from an ethical and a legal perspective. Customer service interactions routinely involve sharing of PII such as addresses and account information. Customers can disclose very sensitive information while on a call with a particularly empathetic customer service representative. And that information often gets stored in ways you don't expect.
At Further, we take privacy protection seriously. We’ve developed custom algorithms to remove PII in the Data Pipeline stage, before it's viewable by Data Scientists or any resultant reports and dashboards. Information that’s specific and unique to an individual interaction, such as PII, isn’t useful from an analytics perspective, anyway. In analysis, we’re looking for trends or cluster groupings to inform our hypotheses that turn into concrete actions to improve business performance.
Activating Insights
Once PII is removed and the data safely anonymized, we can begin building a data store for
isolating trends and developing deep customer understanding as a rich foundation for business improvements. This allows the creation of a real-time feedback loop directly to a customer’s thoughts and feelings, enlightening and enabling opportunities like these:
- Highlight product/service improvements and even new offering ideas.
- Create more intuitive user experiences.
- Enable cost savings through redeployment of customer service resources focused on solving the really difficult problems requiring a professional, empathetic human interaction.
- Unlock important insights validated through better informed marketing campaigns (i.e, A/B testing, optimization, etc.).
- Deliver enhanced understanding and more relevant messaging to customer segments, etc.
- Act as a point of reference to analyze and optimize Interactive Voice Response (IVR).
Natural Language Processing (NLP)
Natural Language Processing allows for analysis of customer interactions. Using tokenization and stemming it extracts the meaning and commonality from sentences and phrases allowing a better understanding of customer experiences. Properly executed NLP not only accounts for the words that were spoken, but it also accounts for phrases and context to appropriately assign a sentiment score. This data set also forms a strong basis for IVR optimizations by highlighting common customer concerns.
Sentiment analysis vs NPS scores
We’ve found that sentiment analysis models can augment customer service surveys or NPS scores that only focus on a subsection of customer interactions and can involve self selection bias. Further’s Sentiment Analysis Models comb through thousands of customer interactions and tag each phrase and the overall interaction as positive, neutral, or negative.
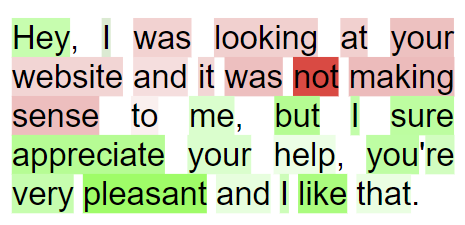
Our models have found that customer sentiment is normally distributed versus customer satisfaction scores (CSAT) or service surveys, which often skew heavily positive. This enhanced granular view is more actionable than a general NPS score because it points directly to the cause of the score rather than an abstract reference. For example, managers could view redacted transcripts of negative interactions with a few simple dashboard clicks.

Creating customer feedback dashboards can be a great way to incorporate these insights into a company’s operating rhythms. A structured way for customer-facing managers to track sentiment, length of calls, key phrases related to strategic initiatives, etc. can democratize improvements to customer experiences. These dashboards, along with the ability to drill into redacted transcripts, can put the voice of customers front and center and set the stage for continuous improvements.
Partnering with Further to craft a real-time customer feedback loop customized to unique business challenges can have wide-ranging effects. It can provide real time feedback on product launches, integration with the Customer Journey analysis, and sync with your Customer Data Platform.
Sentiment to Call Length Correlation
Dashboards tracking customer satisfaction can highlight other important metrics. For instance, the longer the phone call takes, the worse the customer’s sentiment. This makes sense for anyone that’s spent hours on the phone with customer service. But, these sorts of common sense metrics can get lost in the shuffle for companies lacking robust customer satisfaction metrics and dashboards.
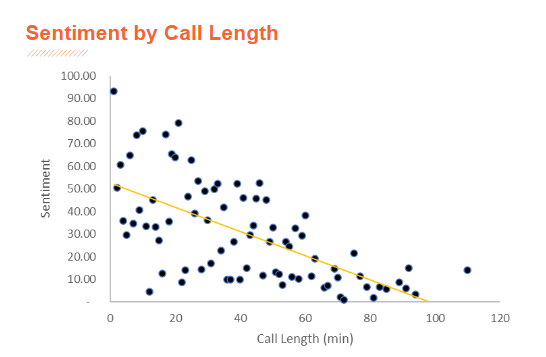
Advanced analytics and AI: Moving at the speed of business
It’s no secret that an increasing pace of change drives business to do more, try new things, and react faster, often in the face of incomplete information. Those companies best able to understand and respond to changing customer needs will succeed, while others fall behind.
But, that’s easier said than done. Working in analytics can sometimes feel like you’re drowning in information overload. In fact, sources agree that the amount of data is growing exponentially and will double every two years. However, overcoming challenges creates opportunities, and those companies best able to unlock the secrets of new data sources will prosper. According to Statica, digitally transformed organizations are forecast to contribute to more than half of global GDP.
A real-time customer feedback loop powered by advanced AI and analytics resulting from safely anonymized customer service data will enable organizations to thrive. It will unlock enhanced new products and service offerings, enable more effective deployment of customer service resources, more effective marketing campaigns, augment customer satisfaction metrics like NPS / customer surveys, enhance segmentation efforts, and help organizations become more flexible and responsive to changing environments and customer needs.
Contact us today to learn how to put your call center data to work! The benefits are many and the results can set your business apart.