Businesses try to use data to improve fact-based decision-making, but many aren’t sure how to best use the overwhelming amount of data at hand. This is the first post in a series that explores data science as a cure for information overload and as a key to data-powered performance.
For most of human history, we’d gather around campfires to discuss our feelings and the day’s challenges. We’d cook and eat together in the ember glow of a dying fire. Under the stars, we’d tell the stories that defined our shared identity. Today we send text messages, eat microwaved leftovers, and post on social media, hoping for approval and validation.
Technology has changed so much in our lives. During the industrial revolution, machines replaced manual labor, changing how we use our bodies for work. And now, in the information age, technologies are changing how we think. This post begins a series examining cognition and how data science technologies will change traditional modes of understanding and decision-making, specifically addressing information overload.

How do you know?
Cognition generates a unified intellectual framework of ideas through synthesizing experience over time. In other words, cognition—the processes by which we learn, remember, and make decisions–constructs a worldview.
For example, we learn how to interact with others through childhood play. We know to share our toys by empathizing with the emotional state of others when another kid steals our toys. We understand how to engage in romantic relationships by observing our parents and watching romantic comedies. We learn how to add and subtract in a classroom by listening to the teacher’s lectures and working through problems in our homework assignments.
Consider a hunter at work 50,000 years ago. He crests a hill and spots a deer drinking water from the creek. Before the deer notices him, he quickly nocks an arrow and shoots it. Bringing the deer back to camp, his tribe rejoices! He feels proud: his friends and relatives praise him as a mighty hunter.
The hunter will probably employ his newly discovered hunting strategy of staying out of sight near a stream on the next hunt. He’ll reason that deer need water; there are a limited number of water sources available; hunting near a water source will provide his best opportunity to get food and approval.
That makes sense. But, the cognitive skills that worked in a hunter-gather society no longer work today, especially in the digital world. We can’t experience the billions of data points or website clicks that mean we’ll bring back the game for our tribe. The serialization of data into ones and zeros does nothing to trigger our senses to help our bodies learn what the data means. We have a wealth of information to drive our decisions, but our brains aren’t up for the everything/everywhere/ all-at-once hunt.
How do you know, today?
Cognitive overload can overwhelm us when we get exposed to more information than our brains can process. Our reactions to cognitive overload range from paralysis to anger and from passivity to motivation to expand our capacity to understand.
But the good news is that we’ve created our way forward. Data science, still in its infancy, has the potential to sort through the avalanche of information and synthesize more coherent intellectual frameworks.
Current approaches to addressing information overload
Today’s approaches primarily focus on summarizing information in more easily digestible forms. Businesses address information overload by creating dashboards summarizing information in graphs or data tables. These dashboards usually allow users to drill down into data and slice and export it into other applications for further analysis.
News aggregation sites summarize headlines and can alleviate information overload to an extent. Others rely on social media’s AI algorithms to summarize points of interest. However, people reliant on algorithms to curate points of attention risk being misled by echo chambers, fake news, or advertisement onslaughts.
Social media’s intent isn’t to inform, but to form addiction, so users spend increasing amounts of time engaging with the algorithm’s biggest game. Clickbait headlines might feed a tribe for a while, but without context and nuance, this sort of cognition breaks down.
In business settings, machine learning to assist decision-making has not become mainstream for several reasons. First, the massive task of moving, transforming, and cleaning data consumes most corporate analytics resources, leaving little bandwidth for more advanced applications. But that will change as technology improves. Those best able to overcome information overload and successfully apply data to business problems will enjoy significant advantages.
Let’s look at a concrete example of how traditional analysis stacks up to the task of handling large data sets. We’ll use a discussion of a popular investment advice book for this. Subsequent posts in this series will contrast the methods used in the book with data science methods.
Large data sets: Traditional analysis
Traditional analysis relies on stitching together a worldview derived from synthesizing experience. One reads books, annual reports, newspaper articles, etc. One makes charts and graphs of data to create visual stories of what’s happening. Christopher Mayer’s book, 100-Baggers: Stocks that Return 100-to-1 and How to Find Them exemplifies such analysis.
Mayer is a guy steeped in the school of value investing. His book outlines the results of his “study” that purportedly enables his readers to identify patterns signaling stocks that will soon experience significant price increases.
He begins his analysis by assembling a database with stock prices and other financial statistics, including return on equity, price to book, price to earnings ratios, etc., from 1962-2014. This was a massive undertaking. After analyzing this database, he identifies 365 stocks that returned 100-to-1 from 1962 to 2014. From this, Mayer claims to have distilled a predictive framework that will signal the 100-baggers of the future.
Unscientific study?
To his credit, Mayer acknowledges the shortcomings of his study, saying:
“There are severe limitations or problems with a study like this. For one thing, I’m only looking at these extreme successes. There is hindsight bias, in that things can look obvious now. And there is survivorship bias, in that other companies may have looked similar at one point but failed to deliver a hundredfold gain. I am aware of these issues and others. They are hard to correct. I had a statistician, a newsletter reader, kindly offer to help. I shared the 100-bagger data with him. He was aghast. […] However, what I’ll present in this book is not a set of statistical inferences, but a set of principles you can use to identify winners. If you’ve read Michael Lewis’s Moneyball, which looks at the principles behind productive baseball players, you know this is a worthwhile exercise.”
I’m not sure how Mayer likens his study to the statistic-heavy approach depicted in Moneyball, since statisticians poo-poo his approach. But, he states his study isn’t scientific, so I applaud his intellectual honesty. A Data Science approach will overcome these limitations, as I’ll show in later essays.
Conventional wisdom
Conventional wisdom seems to be at the heart of Mayer’s book. In traditional cognition, conventional wisdom is built by cyclically perceiving the world, forming impressions, filling in the gaps, interacting with the world, perceiving the world, etc.
Mayer lists conventional value investing wisdom from giants like Benjamin Graham and Warren Buffett. I find his advice sound and his exposition solid. Nonetheless, he’s not saying anything new or connecting these themes to the 100-baggers stocks. He just recites conventional wisdom without providing any data or reason to claim that 100-bagger performances result from these conventions.
These value investing themes strike a chord with experienced investors despite no demonstrable link to the 100-baggers, and set up a giant opportunity for cognitive bias.
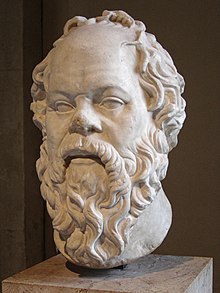
Cognitive bias
Cognitive bias explains how individuals create their own “subjective reality” based on their perception of the world, lending weight to the old adage, “We see the world not as it is, but how we are.” Although this subjective construction of reality—not objective input—may sometimes lead to perceptual distortion and illogical interpretations, it is a solid adaptation in a world with sparse information.
Humans are limited by our sensory input and lifetime. We can only see light in a certain spectrum, hear sounds in a certain range, and we can only remember experiences in the course of a human lifetime. So, the hunter in our example above will never live long enough to go on tens of thousands of hunts. His perception is anchored in his physical body and experiences. However, an ML model can easily “experience” hundreds of thousands of stock price changes or website clicks, and will actually learn more the more data it’s given.
Mayer uses this very human way of cognition (building understanding through his experiences and readings) in an attempt to explain factors that cause 100-bagger performance. Then he describes a set of features (nine or ten characteristics) that purportedly predict 100-bagger stocks. Again, I find these ideas compelling and valuable in and of themselves, but Mayer presents no compelling linkage that this list of conventional wisdom predicts 100-baggers. He’s just an experienced, clearly well-read investor.
In contrast, a data science-informed approach moves business away from subjective interpretation of limited data and perceptual distortions. New technology’s reliance on vast amounts of data drive a fact-based focus to help businesses make better decisions. That doesn’t negate the need to interpret or assign meaning to data. However, it does ground decision-making on broader and richer information than has ever been available before.
Building knowledge using comparisons
The book focuses on the importance of finding companies with an overlap of high Return on Equity (ROE), low price-to-earnings ratio, and sustainable competitive advantages. He compares a sustainable competitive advantage to a castle with a moat. Companies with a sustainable competitive advantage protect their treasure trove of high returns by keeping out competitors with their ‘economic moat.’ I love Mayer’s discussions with others as he tries to find the best approach.
“Jason Donville’s Capital Ideas Fund has been a top performer since inception in 2008. Investing in companies with high and lasting ROEs is the special ingredient that gives his fund such a kick. I called Jason and explained the 100-bagger project and my initial findings. Many 100-baggers enjoyed high ROEs, 15 percent or better in most years. “That’s exactly right, and that’s the kind of stuff we look for,” he said. We fell into discussing his approach and the magic of great-performing stocks.”
He breaks ROE into two main capabilities: investing and financing or capital allocation. He then posits that in order to get outsized returns, one must invest in companies with high ROE’s. Although true, that statement is pretty apparent and lacks insight, in my opinion. Plus, it’s tautology: Naturally, a company generating high returns will pass that along to stockholders. He couples his previous advice with the value investing adage to buy companies with a low price-earnings ratio.
The idea that stocks fall into the intersection of high ROE resulting from sustainable competitive advantage and low multiples seems true. But, it will be interesting to see if these things are predictive or if they’ll crumble in the face of hindsight bias.
Incorporating other biases
Next, he points out that great companies result from great managers. Again, another tautology. But, this idea certainly isn’t predictive. He provides the example of Steve Jobs and Jeff Bezos as great managers, which would predict 100-baggers. That’s undoubtedly true looking in the rearview mirror, but I don’t think anyone would have thought of them as great managers thirty years ago.
Jeff Bezos started Amazon by quitting his job, throwing all his worldly possessions into a U-Haul, and driving cross-country to Seattle to start an online book catalog company. Thousands of dudes quit their jobs to start new companies every year. But, almost none of them revolutionized the retail industry and became the world’s richest man.
Apple and Steve Jobs were the dogs of the 1990s. Steve was fired from the company he founded because he had difficulty getting along with others. Apple struggled for decades. Its comeback with the innovation of the iPod and iPhone took everyone by surprise. Good management is essential, but it’s tough to detect early on. Jobs and Bezos are exceptions to the rule, and it isn’t clear how their success could have been predicted before they rose to fame.
Absence of statistics
Mayer’s study is an excellent example of traditional modes of cognition. He piggybacks on conventional wisdom and thinks back to his successful investing experience. He then applies principles learned to explain 100-bagger performance, just as the deer hunter thought about his success hunting deer.
The human brain collects tidbits of information like these and uses them to triangulate our way to a worldview. Interestingly enough, stochastic gradient descent is the artificial intelligence model most closely aligned to how our brains work. Compare and contrast how Mayer uses his reading and experience to get closer to the truth, as Andrew Ng explains in this video.
Connecting the dots
Mayer’s study is an excellent example of traditional modes of cognition. He piggybacks on conventional wisdom and thinks back to his successful investing experience. He then applies principles learned to explain 100-bagger performance, just as the deer hunter thought about his success hunting deer.
The human brain collects tidbits of information like these and uses them to triangulate our way to a worldview. Interestingly enough, stochastic gradient descent is the artificial intelligence model most closely aligned to how our brains work. Compare and contrast how Mayer uses his reading and experience to get closer to the truth, as Andrew Ng explains in this video.
Large data sets: A data science approach
AI assistance will allow us to overcome information overload and to address, understand, and act upon newly available mountains of data. The next four posts in this series will examine the same problem presented in Mayer’s book—how to pick good stocks—using a data science approach with practical examples. Stay tuned for examples of AI-assisted problem-solving, data ingestion methods and successes, data analysis and study design, and running models and their results.
Read more of our recent Data Science posts:
- How to Reveal a Bot Problem in Social and Display Campaign Tracking
- We’ve All Got ‘Em: Marketing Problems that an RCT Study Can Solve