Harnessing the Future: How Predictive Analytics Shapes Decision-Making
Predictive analytics is the key to forecasting the future and developing strategies based on data, not guesswork.
Discover how to effectively harness this power, the specific techniques involved, and how it’s revolutionizing industries from finance to healthcare. This is your guide through the practicalities of predictive analytics—no fluff, just actionable insights.
Key Takeaways
- Predictive analytics uses statistical techniques and machine learning to forecast future outcomes based on historical and current data, impacting various industries such as finance, healthcare, and retail.
- The process involves collecting high-quality and diverse data, selecting the right predictive model through testing and refinement, and turning insights into actionable strategies to improve efficiency and decision-making.
- Challenges in predictive analytics include ensuring data quality and quantity, addressing ethical issues like privacy and bias, and the need for ongoing adaptation to rapid technological advancements.
Decoding Predictive Analytics
Predictive analytics stands as a significant element within the domain of data analytics, crucial for enterprises to take into account. This approach leverages statistical methods and modeling techniques to anticipate future outcomes by analyzing patterns in current and historical data.
While it’s not akin to having a crystal ball, predictive analytics offers valuable strategic insights applicable across multiple sectors, encompassing:
- Financial trend projection
- Anticipation of machinery breakdowns
- Projections for sales and demand levels
- Analysis of consumer behavior trends
- Identification and thwarting of fraudulent activities
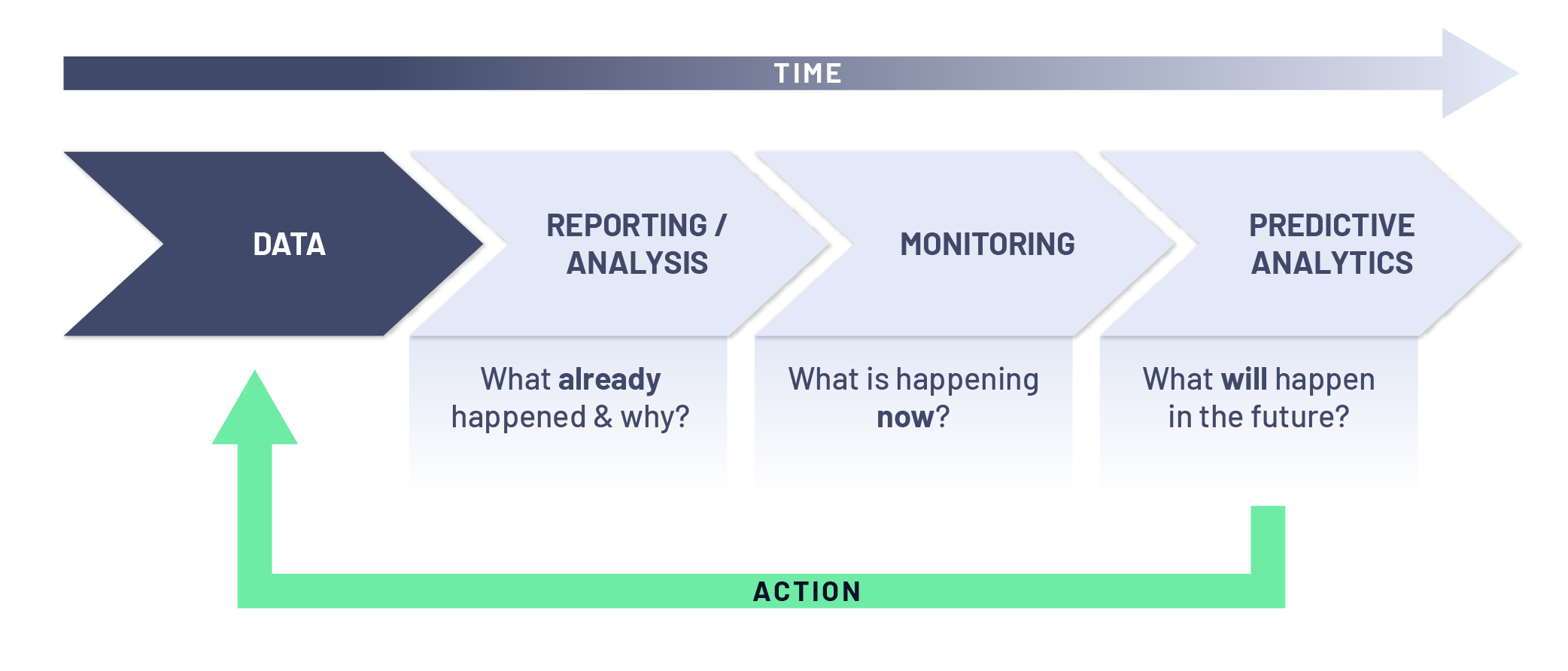
The Essence of Predictive Analytics
Predictive analytics integrates a range of statistical techniques such as modeling, machine learning, and data mining to evaluate present and historical data with the aim of forecasting future or unknown events. Utilizing approaches including regression analysis, decision trees, and neural networks allows for in-depth exploration of information to uncover significant predictions.
Significance of Historical Data
Historical data acts as the crucial foundation for predictive analytics, akin to a rich repository of knowledge that offers essential ingredients to project future trends and facilitate well-informed choices. By utilizing metrics extracted from this historical information—including moving averages, bands, and breakpoints—analysts can predict occurrences such as fluctuations in stock prices with greater accuracy.
Forecasting with Statistical Models
Using statistical models for forecasting is an essential element of predictive analytics. Clustering, for instance, involves organizing data into clusters based on shared characteristics to make predictions about future trends or behaviors using predictive analytics models.
Incorporating this clustered information, time series modeling is applied to predict the timing of specific occurrences, like when surges in sales or increased demands on customer service might happen.
The Mechanics of Predictive Analysis
Predictive analysis entails a series of stages that start with gathering and classifying data from diverse origins to forecast future trends. This involves processing the data through cleansing, conversion, and encoding steps to prepare it for analytical examination.

Gathering and Preparing Data
The process of data collection for predictive analytics encompasses accumulating both structured and unstructured data from a variety of sources, including transaction records, customer databases, social media platforms, and website traffic metrics. To guarantee the integrity and dependability of this collected information, it undergoes a purification stage where preprocessing measures such as deduplication, error rectification, and standardization of formats are applied.
Selecting the Right Model
Understanding the data, problem at hand, and objectives is essential for selecting an appropriate predictive model. This process does not have a universal solution. Rather it necessitates tailored experimentation.
To evaluate their effectiveness, various models undergo backtesting using historical data. Following this assessment, the best-performing model is chosen for implementation.
From Analysis to Action
As businesses leverage the power of predictive analytics to forecast demand and discern peak service times, they are able to more effectively distribute their resources. With the escalation in data volume comes an imperative need for investment in infrastructure that can manage the augmented data loads and enhance data processing to preserve efficiency.
AI and Predictive Analytics: A Power Duo
Artificial intelligence (AI) has significantly propelled the evolution of predictive analytics, taking it to new heights. By streamlining intricate procedures and recognizing patterns that surpass what humans can discern, AI broadens the potential of predictive analytics profoundly.
This potent combination doesn’t just improve outcomes, it revolutionizes the modus operandi and decision-making processes within organizations.

Machine Learning's Role in Predictive Modeling
Leveraging historical data, machine learning algorithms improve the accuracy of predictive analytics when making assumptions about future events. The refinement process for enhancing a model’s precision and accuracy involves testing it on new, unseen data to ensure better predictive performance in machine learning applications.
Analyzing Complex Data with AI
In data analysis, AI stands unparalleled. Using machine learning algorithms, AI can identify intricate patterns in complex datasets with minimal human oversight. It's like having an exceptionally capable data analyst working tirelessly around the clock!
AI-Driven Predictive Insights
The implementation of AI for predictive insights has the potential to transform how businesses operate. Consider these applications of artificial intelligence:
- Anticipating and fulfilling client demands in advance
- Refining the management of inventory quantities
- Providing tailored interactions for customers
- Boosting the effectiveness of supply chain operations
Real-World Applications of Predictive Analytics
Practical rather than merely theoretical, predictive analytics serves as a valuable instrument employed in diverse sectors such as finance and healthcare to guide and improve strategic decisions.
Boosting Sales with Predictive Analytics
In retail, predictive analytics has revolutionized the way businesses operate. By implementing this technology, retailers are empowered to:
- Forecast product demand with precision
- Adjust pricing strategies in accordance with consumer purchasing patterns
- Enhance their sales performance substantially
- Successfully maintain a loyal customer base
Enhancing Healthcare Delivery
Predictive analytics is revolutionizing the way services are provided in healthcare, too. Its transformative impact is evident through:
- The customization of treatment protocols to fit individual needs
- Early identification of personal health risks for proactive care
- Assisting healthcare experts with accurate disease diagnosis
- Identifying likely future medical concerns before they arise
Streamlining Financial Services
Predictive analytics is also transforming the financial landscape by analyzing transactions to detect fraud, evaluating loan risks, and projecting stock market prices. The sector heavily relies on this technology for various aspects of finance management.
Achieving Competitive Advantage with Predictive Analytics
Utilizing predictive analytics can do more than just improve operations. It offers a competitive edge by leveraging its capabilities in predicting outcomes and trends.
Retaining Valuable Customers
Predictive analytics excels in the crucial domain of customer retention. Through forecasting client requirements, tailoring interactions, and engaging with them efficiently, companies are able to keep their valuable customers.
Optimizing Marketing Campaigns
The utilization of predictive analytics in marketing is crucial for companies as it serves several purposes.
- It deciphers consumer behavior.
- It guides the formation of well-founded marketing tactics.
- Predicts upcoming trends and customer inclinations.
- Assists in designing focused advertising campaigns.
- Augments the customization aspect of marketing initiatives.
- Concentrates on prospects with a high likelihood to convert.
By harnessing predictive analytics, firms can enhance their promotional activities by identifying the most effective strategies to use.
Resource Management and Planning
Predictive analytics can enhance operations in resource management and planning by efficiently allocating resources. Utilizing predictive models to forecast customer demand allows businesses to bolster efficiency and safeguard valuable assets.
The Journey of Data Science: Predictive Analytics Techniques
Within the expansive realm of data science, techniques like decision trees and neural networks, along with regression methods, are harnessed for predictive analytics—analyzing information to forecast future events.
Data Mining Techniques
Techniques involved in data mining play an essential role within the scope of predictive analytics. This process includes collecting, purifying, and scrutinizing data to detect trends that can be used for forecasting purposes.
Regression Techniques and Their Uses
Techniques of regression, such as polynomial and logistic regression, serve to establish the connection among variables while forecasting results. They introduce statistical precision into the chaotic nature of events in reality.
Neural Networks and Advanced Analysis
Mimicking the functions of the brain, neural networks enhance predictive analytics by employing advanced analysis methods. They are capable of identifying patterns within historical or incomplete data sets, thus facilitating smart forecasts regarding future outcomes.
Overcoming Challenges in Predictive Analytics
Several hurdles, including challenges in data collection and rapidly changing technology, can impede the successful deployment of predictive analytics.
Data Quality and Quantity
It is essential to secure data that is both substantial and of high quality for successful predictive analytics. Challenges such as consent and privacy concerns, along with issues like missing values or disparate formats, have the potential to undermine both the volume and integrity of the data.
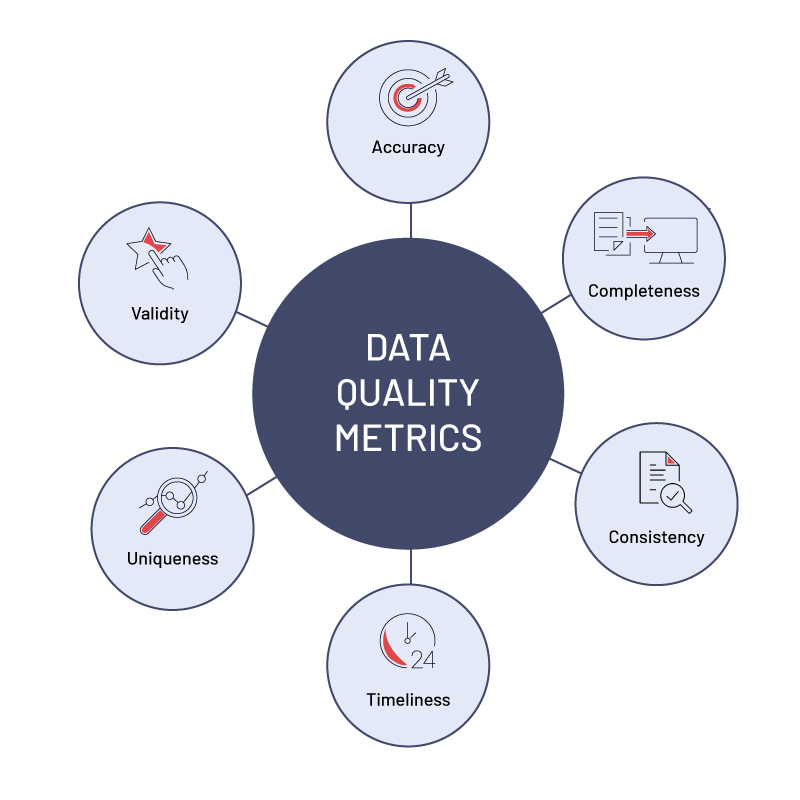
Ethical Considerations and Bias
Ensuring data privacy, minimizing bias, maintaining transparency, and ensuring accountability are critical factors for the responsible use of predictive analytics, which also brings with it ethical considerations.
Staying Ahead of Technological Changes
In the fast-evolving field of predictive analytics, staying ahead of technological advancements is crucial. This requires a commitment to continuous learning, professional development, and a flexible mindset to keep up with ever-changing technologies.
Summary
In summary, predictive analytics is a powerful tool that uses historical data and machine learning to forecast future outcomes. While it has its challenges, the benefits are substantial, providing businesses with essential insights for informed strategy development in various industries.
Frequently Asked Questions
What is predictive analytics?
Utilizing statistical methods and modeling approaches, predictive analytics aims to anticipate future outcomes by examining patterns in current and historical data.
This process is instrumental in predicting upcoming trends and behavioral patterns.
How does predictive analytics work?
By gathering and analyzing data, predictive analytics employs a suitable model to forecast outcomes.
How is AI used in predictive analytics?
Predictive analytics has its capabilities enhanced by the use of AI, which not only automates intricate processes but also detects patterns that surpass human abilities.
What are some real-world applications of predictive analytics?
Industries such as finance, healthcare, and marketing extensively employ predictive analytics to enhance strategic decision-making.
What are some challenges in implementing predictive analytics?
Deploying predictive analytics successfully can be challenging due to several obstacles. These include the complexities of collecting, storing, and processing data, a scarcity of specialized knowledge, and constantly changing technology. These factors can hinder the effective application of predictive analytics initiatives.